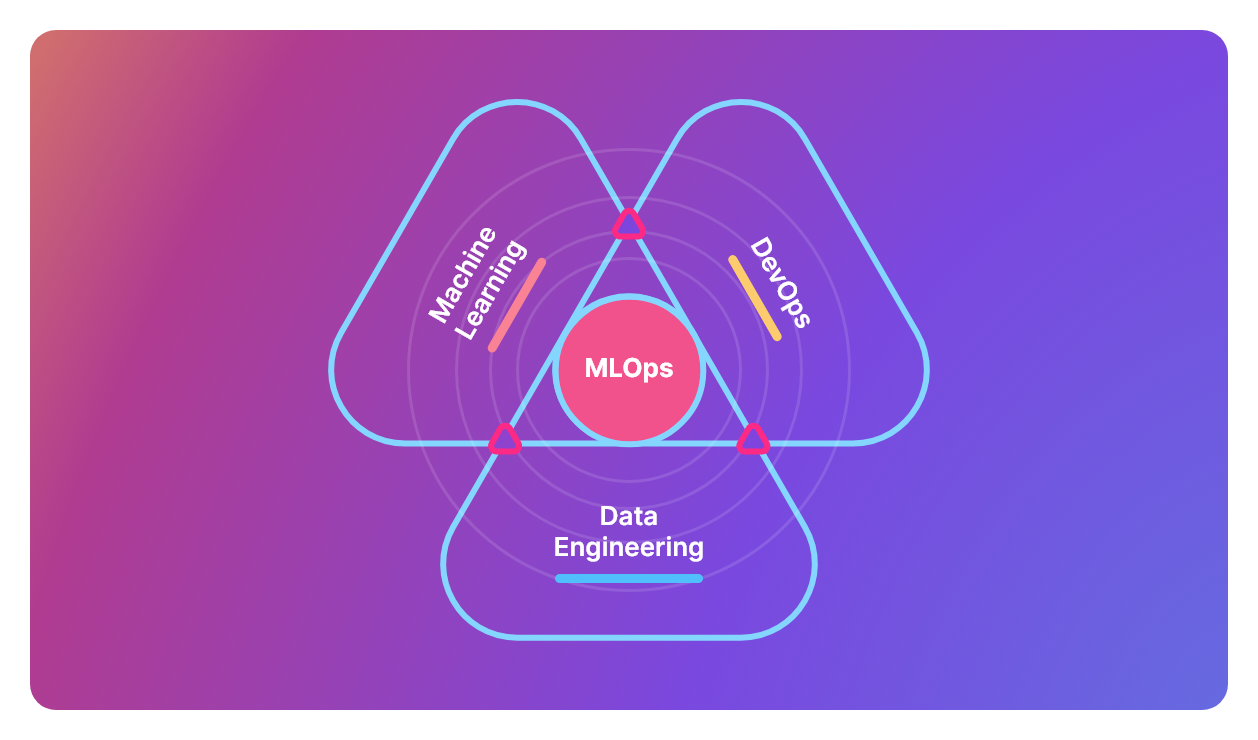
Building blocks of MLOps: What, Why and How?
.webp)
MLOps has become the centre of focus for any ML team to scale since 2020. There are approx 284 tools created in MLOps. So what is MLOps? Why do we need MLOps?
What is MLOps?
The goal of MLOps is to reduce the technical friction to get the model from an idea to production in the shortest possible time to market with as little risk as possible.
MLOps is the set of practices at the intersection of Machine Learning, DevOps, and Data Engineering.
Why MLOps?
Typical machine learning project flow looks like this: There is a data scientist who needs to solve some business problem that can provide some impact. The data scientist investigates the problem, gets the data, does data analysis, cleans the data, experiments with different kinds of models, and then saves the best model and the data used for the creation of that model. Now the model needs to be served so that it can be consumed by the business applications.
What can go wrong?
- The number of requests can go high that a single server might not be able to handle.
- The underlying data can change which can affect the model predictions.
- The model might not perform best in all cases, so re-training might be required.
- The business problems can change which leads to either modification of the model or the addition of new models..
and many more...
How MLOps help?
MLOps helps in enforcing certain practices which help in reducing risks. It can help in Shorter development cycles. Better collaboration between teams. Increased reliability, performance, scalability, and security of ML systems and more...
Industrial Surveys
- 72% of a cohort of organizations that began AI pilots before 2019 have not been able to deploy even a single application in production.
- Algorithmia’s survey of the state of enterprise machine learning found that 55% of companies surveyed have not deployed an ML model. i.e 1 in 2 companies.
Successful deployments, adapting to changes, and effective error handlings are the bottlenecks for getting value from AI.
Model Development
Data Engineering
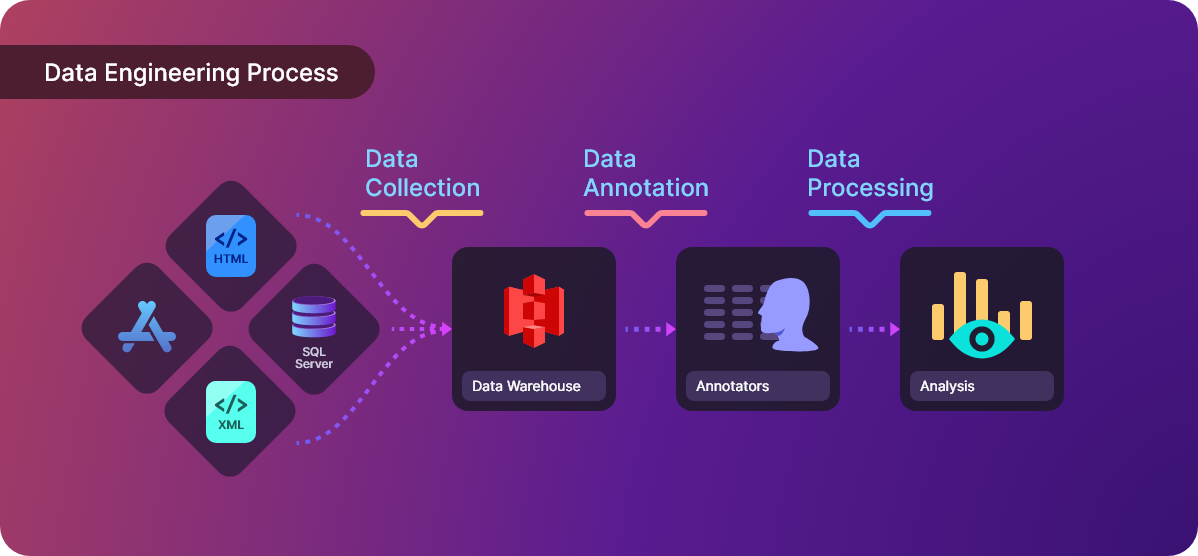
Data Collection: Data is needed for developing a model. Data can come from different places like data storage, fetching the data lively from apps, etc. There could be cases where the data is not sufficient. So data needs to be generated either manually or programmatically in these cases.
Data Annotation: Data might not have been labeled all the time. At times, the labels assigned to the data might not be accurate. In either of the cases, data needs to be annotated (or) validated.
Data Processing: Depending on the problem, data needs to be processed so that it would be useful for model creation. Feature engineering needs to be done to identify useful features. Data visualizations might be needed for a better understanding of the characteristics of the data.
Model Engineering

Modeling: This is the part that everyone is aware of. There are many kinds of models available. It could be statistical models, machine learning algorithms, or deep learning models. There are different kinds of modeling techniques that are also available like supervised learning, unsupervised learning, and semi-supervised learning techniques.
Hyper-parameter tuning: Each model has its own parameters and some modeling parameters. Fine-tuning them will lead to a better model. Some examples include hidden size, number of layers, batch size, learning rate, loss functions, etc.
Model Validation: Choosing the right metric is a crucial part of model engineering. Once the metric is finalized, trained models need to be validated based on this metric. Data used for validation should not be present in training. Unsupervised approaches might not have the training, so all data can be used there.
Model Packaging: Developed model might not be used in the same environment. The deployed environment might not have the required dependencies or run in a different hardware setup. Model packaging will help in resolving these kinds of issues. Some examples like docker container, ONNX, pkl will be used.
Model Deployment
Once the model is developed, it needs to be deployed so that it can be used in real-time.
Deployment Methods
Online vs Batch Inferencing
Some problems might require immediate predictions from the model (ex: conversational agent, self-driving car). Some others might not need immediate predictions and it’s okay having some delay (Feedback analysis).
Auto-Scaling
Scaling is a core part of the deployment, where systems are scaled according to the number of requests. There are two kinds of scale:
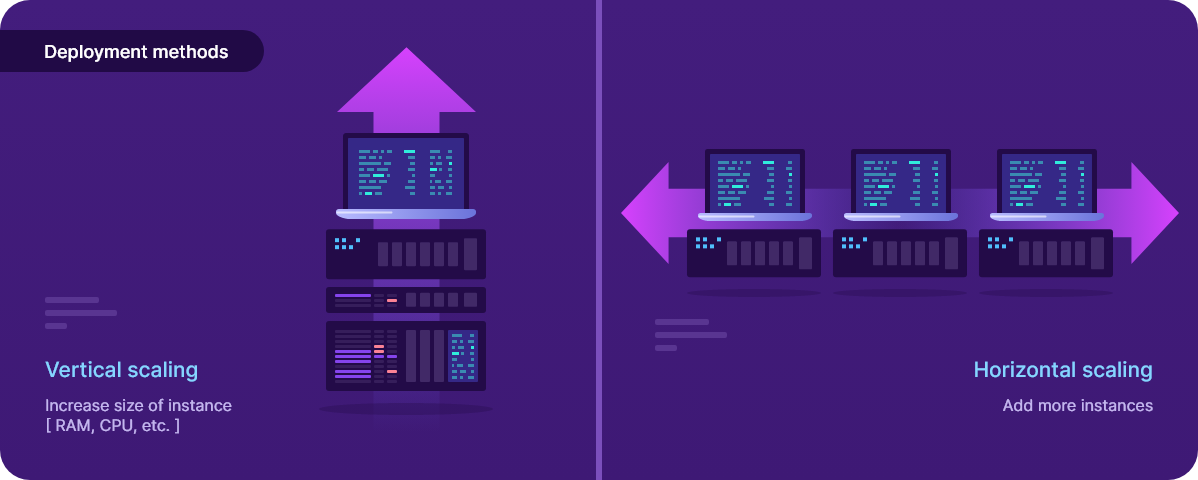
Vertical scaling means replacing the same compute instances with larger more powerful machines.
Horizontal scaling means replacing with more or less a number of the same machines.
Auto-scaling can be achieved in multiple ways: Kubernetes-based scaling, Serverless (Lambda) based scaling.
Deployment Stages
Once the model is developed, we should not directly update it in production. It should be done in stages, so that we can have the flexibility to catch any errors, to compare the performance w.r.t model that is in production, the update should be smooth so that end-user does not feel any downtime, etc.
To tackle this, deployment is usually done in stages.
Staging
First, the model is deployed to staging and all the sanity checks are performed here like latency, outputs are coming in the expected format, validation metrics are coming same, scaling accordingly to the requests, etc.
Production
Model can be deployed in production in different ways.
In the progressive delivery approach, a new model candidate does not immediately replace the previous version. Instead, after the new model is deployed to production, it runs in parallel to the previous version. A subset of data is redirected to the new model in stages (canary deployment, Blue/green deployment, etc). Based on the outcome and performance of the subset, it can be decided whether the model can be fully released and can replace the previous version.
Blue/Green Deployment
Blue-green deployment is a deployment strategy that utilizes two identical environments, a blue and a green environment with different versions of an application or service. User traffic is shifted from the blue environment to the green environment once new changes have been tested and accepted within the blue environment.
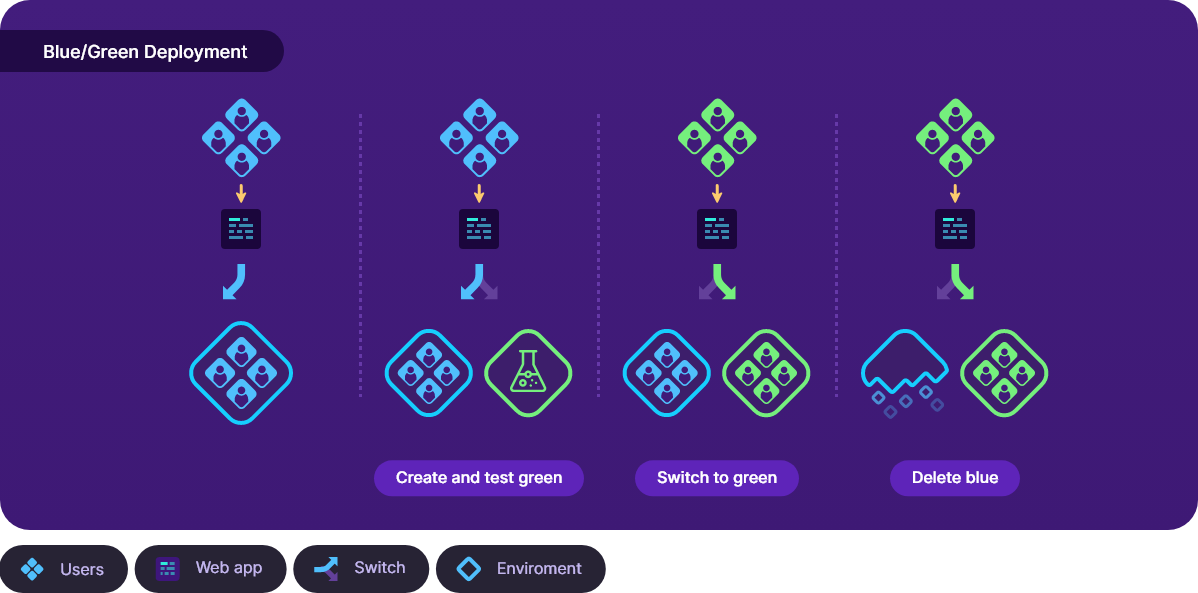
Canary Deployment
Canary deployment is a deployment strategy that releases an application or service incrementally to a subset of users. All infrastructure in a target environment is updated in small phases (e.g: 2%, 25%, 75%, 100%).
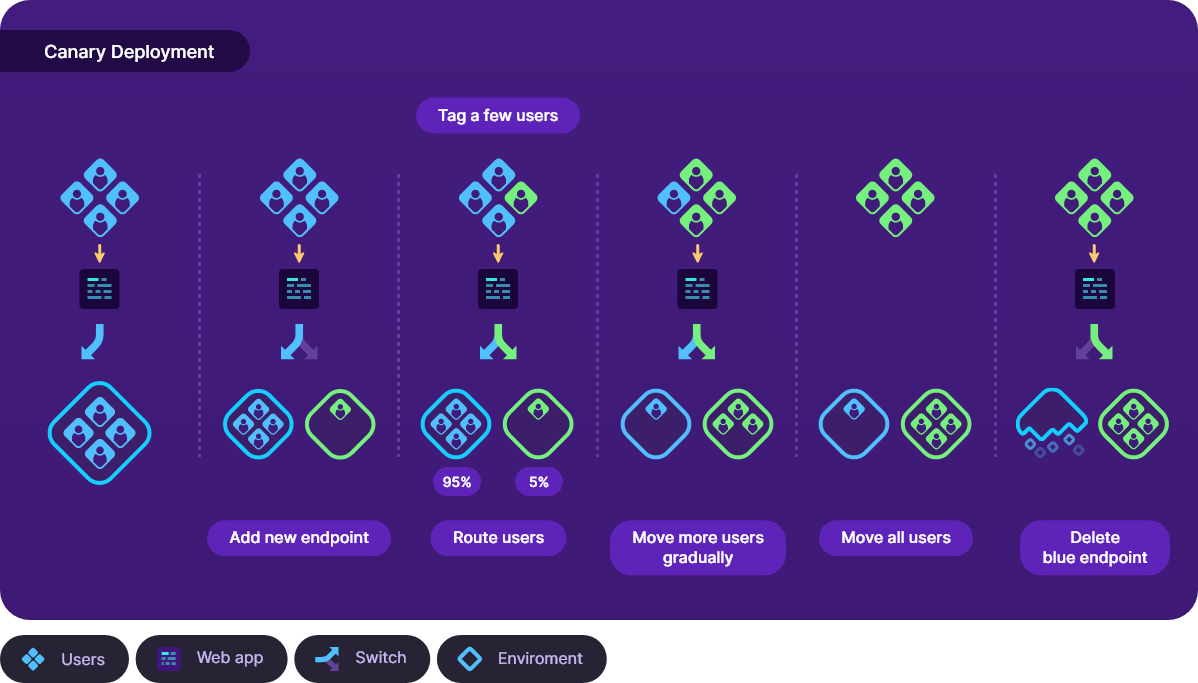
The model can be directly replaced in production without all these steps also depending on the use-case.
Monitoring
Model deployment is not the end of development. It’s only halfway. How do we know that the deployed model is performing as expected?
Monitoring systems can help give us confidence that our systems are running smoothly and, in the event of a system failure, can quickly provide appropriate context when diagnosing the root cause.
Things we want to monitor during training and inference are different. During training, we are concerned about whether the loss is decreasing or not, whether the model is overfitting, etc. But, during inference, We like to have confidence that our model is making correct predictions.
What to monitor?
Performance Metrics:
Response time: This metric is useful in understanding the time taken for the request to get completed.
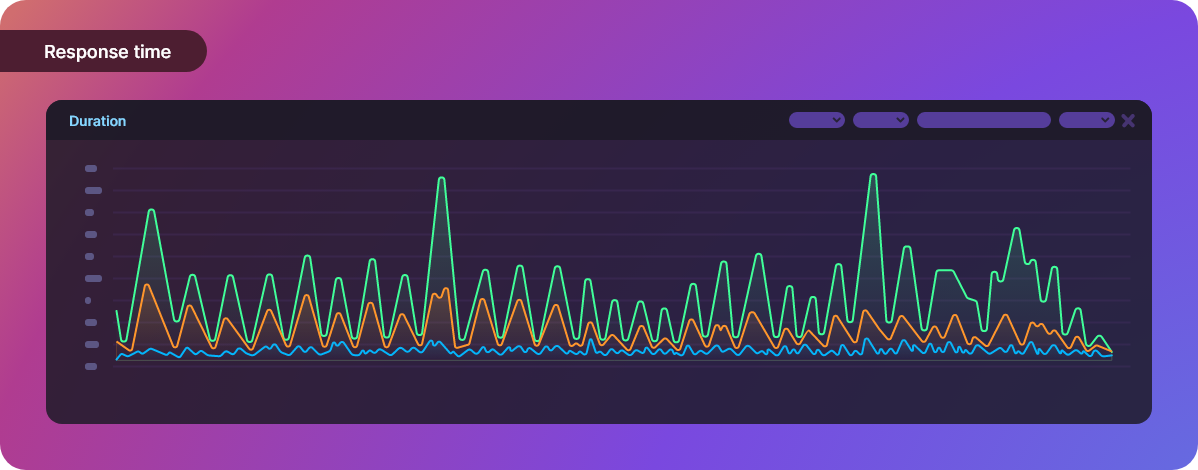
Monitoring this metric will help in understanding when requests are taking higher / lesser times. Alerts can be created on top of this, to get notified when there is an abnormality in response times.
Resource Utilisation: This metric is useful in monitoring CPU/GPU/Memory usage.

Monitoring this metric will help in understanding how the resources are being used. Are the resources being used to their capacity? Alerts can be configured to get notified when the resources are under-utilized or over-utilized upon a specific value.
Invocations: This metric is useful for monitoring how many times a service a called.
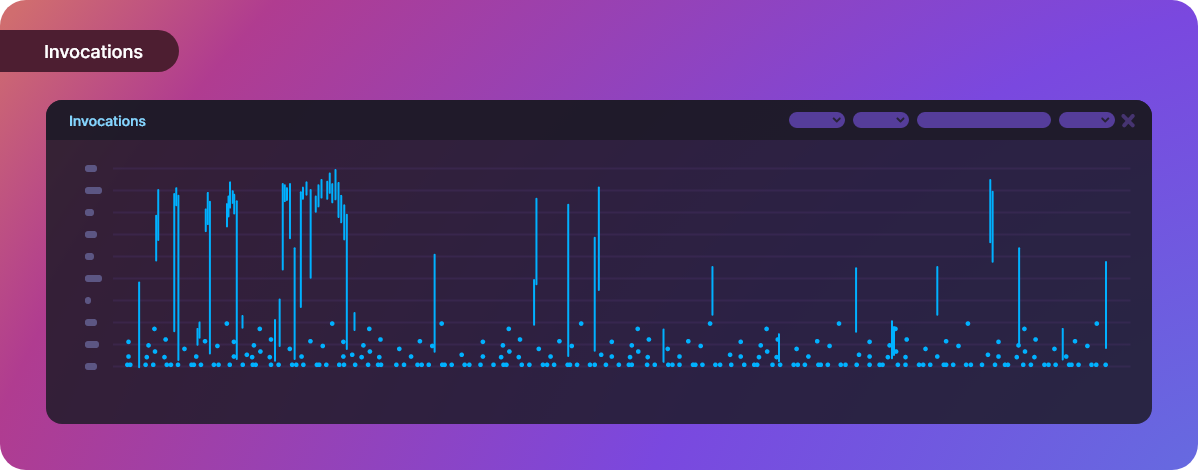
There could be different services (or) different methods in a single service. Monitoring how many times either a service/method is invoked will help in understanding are the services are being called as expected. In the case of auto-scaling, this metric will help in understanding, how many lambdas, containers, pods, etc are invoked.
Success and Error rates: This metric is useful in monitoring how many requests are executed.

Not every request will be successful. There could be errors, timeouts, etc could be happening due to various issues. This metric will help in understanding how the system is performing overall like how many requests are successful and how many failed.
Data Metrics:
The major variable in ML systems is the data. Monitoring data will help in understanding if there are any data leaks, data distributions, etc. Let’s see some metrics which are used for these.
Label distribution: This metric is useful in monitoring the number of predictions made for a label.
There could be no predictions made for some labels. Inversely, the model could be predicting only some labels all the time. Having this metric will help in understanding how the label predictions are distributed in run time. And alerts can be configured if there is a surge/drop-in label counts → Anomalies.
Data drift is one of the top reasons model performance degrades over time. For machine learning models, data drift is the change in model input data that leads to model performance degradation. Monitoring data drift helps detect these model performance issues.
There are different cases where a data drift can occur depending on the use case. Some of the popular ones are:
- Covariate Shift: This happens when the distribution of input data shifts between the training environment and inference environment. Although the input distribution may change, the output distribution or labels remain the same. Statisticians call this covariate shift because the problem arises due to a shift in the distribution of the covariates (features).
Example:
For a cat-dog classification problem, let’s say training is happened using real images. During inference time, if the input is animated images, still the output does not change. So though the input distribution changes (real → animated), the output distribution (cat/dog) is not changed.
Monitoring this will help in improving the model performance.
- Label Shift: This happens when the output distribution changes but for a given output, the input distribution stays the same.
Example:
Say we build a classifier using data gathered in June to predict the probability that a patient has COVID19 based on the severity of their symptoms. At the time we train our classifier, covid positivity was at a comparatively lower rate in the community. Now it’s November, and the rate of covid positivity has increased considerably. Is it still a good idea to use our classifier from June to predict who has covid?
Monitoring this will help us in understanding the changes in output distribution which lead to the re-training model.
Covariate shift is when the input distribution changes. When the input distribution changes, the output distribution also changes, resulting in both covariate shift and label shift happening at the same time.
- Concept Drift: This is commonly referred to as the same input → different output i.e when the input distribution remains the same but the conditional distribution of the output given an input changes.
Example:
Before COVID-19, a 3 bedroom apartment in San Francisco could cost $2,000,000. However, at the beginning of COVID-19, many people left San Francisco, so the same house would cost only $1,500,000. So even though the distribution of house features remains the same, the conditional distribution of the price of a house given its features has changed.
Concept drift is a major challenge for machine learning deployment and development, as in some cases the model is at risk of becoming completely obsolete over time. Monitoring this metric will help in updating the model with respect to data changes.
Monitoring systems can help give us confidence that our systems are running smoothly and, in the event of a system failure, can quickly provide appropriate context when diagnosing the root cause.
Artifact Management
One of the major differences different between ML and others is data and models. The size of data, and models can vary from a few MB to TB. Having a well-defined Artefact management will help in auditability, traceability, and compliance, as well as for shareability, reusability, and discoverability of ML assets.
Feature Management: This allows to share, re-use & discover features and create more effective machine learning pipelines. Maintaining features in a single place, will help in speeding up experiments and also inference times → no need to compute features from scratch, read the already computed ones and run the pipelines.
Dataset Management: There could be different versions of data, different splits of data, data from different sources, etc. Having dataset management in place ensures that the schema of the data will be constant. Being able to access any of the combinations at a later point in time will help in reproducibility and lineage tracking.
Model Management: There could be a number of models in production at scale, and it becomes difficult to keep track of all of them manually. Having model management helps in tracking. We should be able to lineage tracking on a model → what data is used, what configurations are used, and what is the performance on validation/test datasets? It should also help in the health checks of the models.
Summary
Machine Learning will enable the business to solve use cases that were previously impossible to solve. Delivering business value through ML is not only about building the best ML model. It is also about:
- Able to experiment, train, and evaluate models at ease
- Building systems that are replicable
- Serving the model for predictions that can scale according to the usage
- Monitoring the model performance
- Tracking and Versioning datasets, model metadata, and artifacts
Though it is not required to have all the components, as it can vary from organization to organization, having a reliable robust MLOps system will lead to stable productionising of ML models and reduce sudden surprises.