
Manually Tagging Customer Feedback is Ridiculous
.webp)
It’s your 38th support ticket of the day. This one has been open for 5 days now. There has been a considerable back and forth, and you’ve had to get input from the engineering team as well. Finally, the customer confirms that the issue is resolved and thanks you for your help. While the overall resolution time on this one was high, you did well on the other two metrics - time to first response, and the CSAT score. You heave a sigh of relief, and excitedly move your mouse to hit ‘Close Ticket’.
But just then, a familiar horror strikes you – “I have to apply tags for this multi-day support interaction so that the product team can learn from it”. “Why can't my seemingly smart product team figure this out for themselves?”, you wonder for a moment. You catch yourself from wondering too much since there are a dozen more tickets left in your queue.
You open the tags dropdown and scroll through the utterly random and fast-growing assortment of 700+ support tags and select the two which makes the most sense. Time for the 39th ticket.
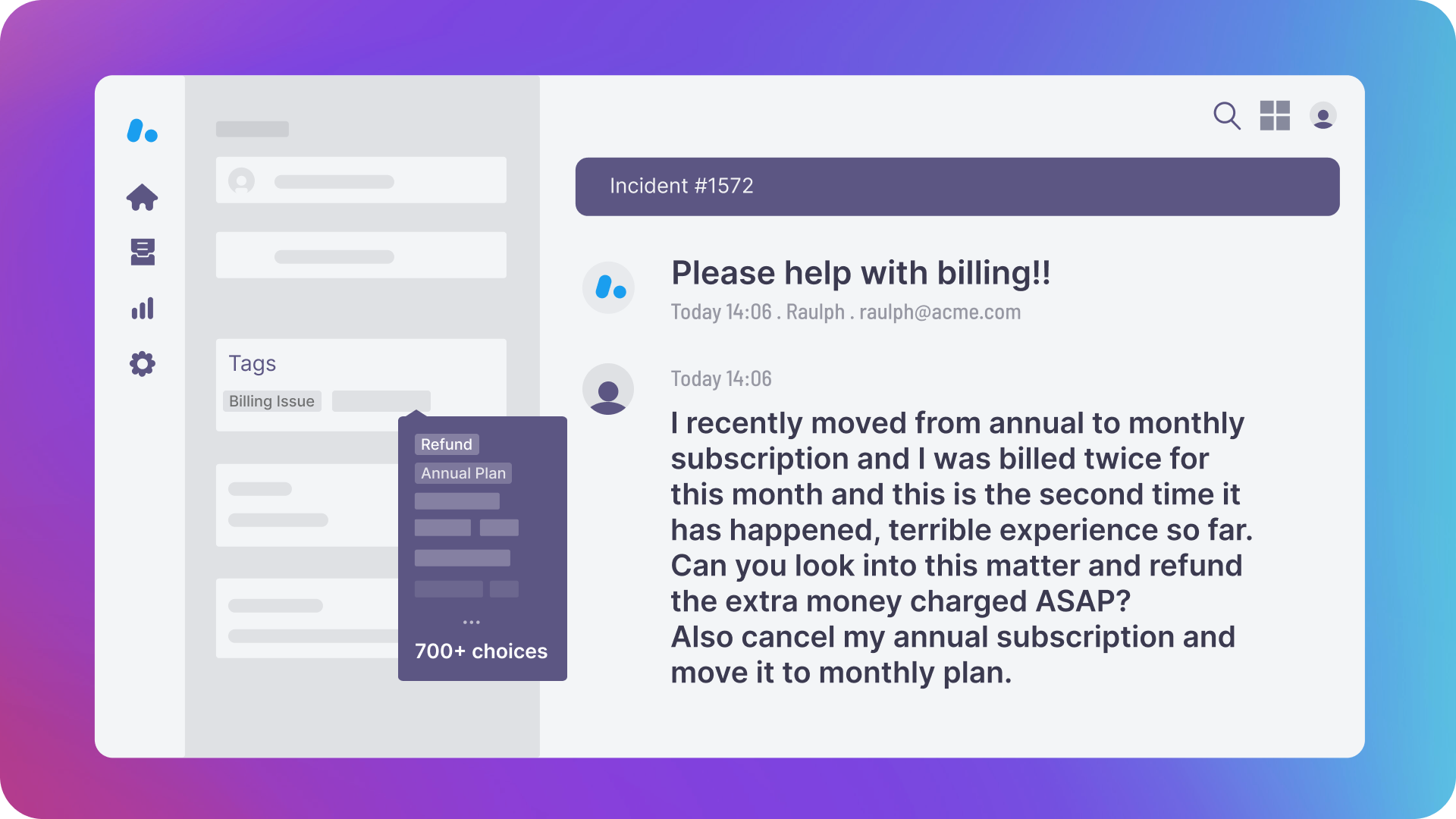
It's 2024, and this is still how 95%+ product orgs learn from their customer feedback. Multiple folks have written about the effort and the process to create a robust feedback taxonomy and maintain it. Initiatives like these are helpful to stay close to customer pain, and can work at lower feedback volumes. However, manual tagging falls apart spectacularly once things start to scale, and customer feedback is coming from multiple channels.
Fareed Mosavat brilliantly summarized this for me from his experience leading product and growth at companies like Slack and Instacart.
"Qualitative data is a critical input in product decision-making. Unfortunately, product teams can't even use the data most of the time because of how inaccurate the manual tagging is. If somehow, you do come around to the data trust factor, then you are faced with high-level tags like 'performance issues' or 'billing'. These are good to know but can't be acted upon in any meaningful manner since there is no way to dive any deeper actually to extract granular insights from it." - Fareed Mosavat, Chief Development Officer, Reforge
Here are the four most common systemic flaws we see in manually tagged customer feedback for fast scaling companies.
Why manually tagging customer feedback doesn’t work
1. Inconsistent across all feedback sources
Each feedback source has its own taxonomy, if there is even the effort to tag all sources in the first place. Consequently, your Gong.io call recordings have a completely different set of tags compared to what your Sales Engineers are using in Salesforce notes, to how your social media team categorizes Twitter feedback, to how your support team tags Zendesk tickets.
What exactly are you learning from?
.png)
2. Grossly Inaccurate
Dozens of humans quickly selecting from an ever growing list of 500+ tags, and applying them. What else were you expecting? The surprising part is not that there is inaccuracy, but the magnitude of it.
I’ve never seen any company have a greater than 60% accuracy in manually applied tags, something that all major stakeholders are already aware of.
This lack of trust means that qualitative data, a potentially invaluable source of customer insights, goes unused.
3. Tags that are too broad and hence in-actionable
Tagging broad topics like ‘Billing - refund’ and ‘Mobile - performance’ is helpful for a 1000-foot view of the topics of customer feedback, but misses all the in-depth context and granular pain that product development teams crave to learn to drive product improvements.
4. Feedback taxonomy that can’t adapt and scale with your product
Most manual tagging endeavors start with a relatively small list of a 15-20 tags, which explode over time by up to 50X as demand for granularity increases, and as product teams continuously ship new features and improvements.
Consequently, there is no proper thought put into the best way to organize your customer feedback taxonomy, and each set of new tags with every feature launch is just added to the list as an afterthought.
By the time this becomes a major pain for the product team, and you think of recreating and re-setting your feedback taxonomy, you’re already sitting on a corpus of about half a million pieces of feedback. What are you going to do, re-tag all of it manually?
If it so flawed, why is everyone still manually tagging as much customer feedback as they can?
Because learning from customer pain is the most important input in building a long-term successful product.
Flying blind on building products is a death knell. So, despite it being so painful and fundamentally flawed, almost all product development orgs go through this ordeal of learning and scaling manual tagging to give them the best shot of their product’s success.
So, how do you set up an effective feedback taxonomy that scales well over time? You need automation to counteract the major systemic flaws of learning from manual tagged customer feedback. But just randomly plugging in GPT-3 or Google Natural Language API isn’t going to solve any of the aforementioned systemic flaws. Here's how to do it correctly.
How to set up an effective automated customer feedback taxonomy
Here are the key requirements that you need to set up your automated feedback taxonomy for success.
1. Customized and fine-tuned to your product’s terminology
Is it ‘zoom’ as in ‘I can’t zoom in on the image’ or ‘zoom’ as in ‘we need a Zoom integration’. These details matter a lot. Which is why just plugging an off the shelf NLP system like GPT-3 or AWS Comprehend is a terrible idea.
Any Machine Learning you use to automate feedback tagging absolutely has to be customized and fine-tuned to your company and product.
2. Granular enough to make it actionable
“There were 100 more mentions of dark mode this month compared to last month.” Okay, thank you. But we already have a dark mode. So, what exactly is the feedback and who’s asking for it? Now compare this to: “Since launch of the new beta UX, there is a 40% increase in users saying that the dark mode on Android does not work properly.”
This is actionable and gives you enough context into the user pain. Just broad topics won’t be actionable. Your feedback system needs to be able to identify and categorize granular and precise reasons from the raw feedback.
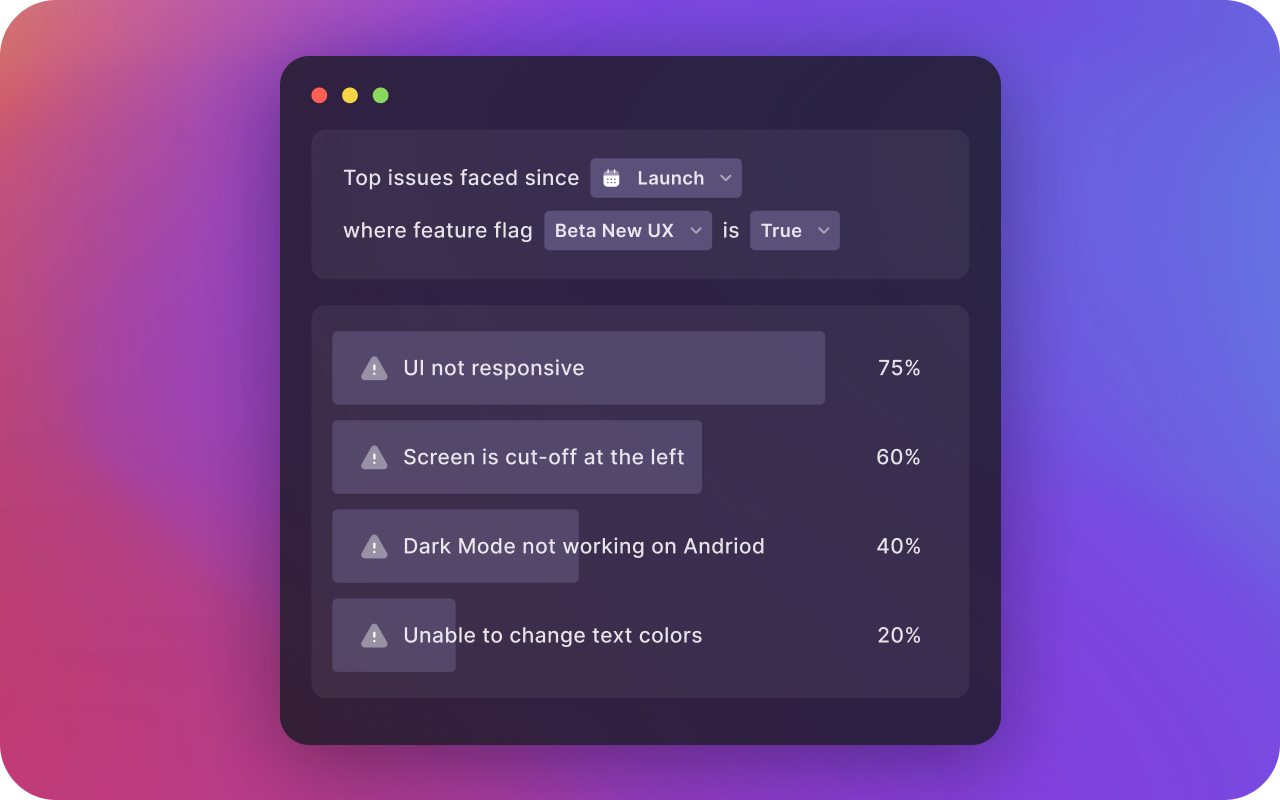
3. Consistent across all sources and languages
Imagine having analytics only on your Android app, and intentionally not tracking iOS and web users. You wouldn’t do that, right? So, why only learn from just the feedback of one or two sources, and intentionally leave out all others?
It is critical that the feedback taxonomy you have is consistently applied across all sources of feedback and across all languages. What you see as 20 complaints on the new sync issues, might actually be 200, which may completely change how you think about its prioritization.
4. Updates automatically as your product changes
The best product development teams are always learning, experimenting, and shipping. Your feedback taxonomy needs to be a living thing that can adapt and automatically update every few weeks to accommodate for new feedback patterns as well as new feature launches.
5. Backwards compatible and re-organizable
Hypergrowth teams periodically evaluate how their product pods are structured, and which product areas are under whose purview. It is important for the feedback taxonomy to be flexible to easily adapt for such changes, and update all historically applied feedback tags accordingly.
FAQ
Question: How does Enterpret's automated feedback taxonomy solution compare to other existing automated tagging systems, and what sets it apart in terms of effectiveness and accuracy?
Answer:Enterpret's automated feedback taxonomy solution distinguishes itself by focusing on customization and fine-tuning to each company's specific terminology and product context. Unlike off-the-shelf NLP systems, Enterpret emphasizes the need for tailored ML models that accurately capture the nuances of customer feedback. This customization ensures that the system can precisely categorize feedback and provide actionable insights that align with the company's objectives and challenges.
Question: Can you provide concrete examples or case studies of companies that have successfully implemented Enterpret's automated feedback taxonomies ans highlight the impact it had on their product development processes and outcomes?
Answer: Many of our customers are finding practical implementations of AI to avoid manually tagging feedback to unlock impressive use cases and outcomes across diverse industries and product types. These case studies highlight key metrics such as improved response times, increased customer satisfaction scores, and more informed product decisions resulting from the implementation of automated feedback taxonomies:
- How Notion is supercharging its product feedback loop using Enterpret
- How Apollo.io grew 9x by mapping customer feedback to revenue
- How Figma leverages AI to scale its product feedback loops
- How Feeld uses Enterpret to actively listen to community feedback during a product launch
Question: What challenges or limitations might arise when transitioning from manual to automated feedback tagging, particularly in terms of implementation, integration with existing systems, and user adoption within product teams?
Answer: Transitioning from manual to automated feedback tagging presents several challenges that companies need to address. These may include technical hurdles related to integrating the automated tagging system with existing feedback collection platforms and workflows. Moreover, ensuring user adoption within product teams requires effective training and communication to demonstrate the benefits of the new system and address any concerns about reliability and accuracy. Additionally, companies may need to allocate resources for ongoing maintenance and optimization of the automated taxonomy to ensure its continued relevance and effectiveness as the product evolves.
Are you still trying to learn from manually tagged feedback?
What has your company tried to implement in order to learn more effectively from customer feedback? Anything that has worked well, or not?
At Enterpret, we have put a lot of thought and effort into solving for all 5 of these required capabilities for product teams like Notion, Loom, Descript, The Browser Company and Ironclad. We collect your raw customer feedback wherever it lives and create a machine learning model customized to your product to automatically categorize feedback into themes and reasons. Layer that with easy-to-use analytics so that you can prioritize effectively and ensure you’re working on the highest impact customer problems.
If you’d like to share your story or learn more, reach out at namaste@enterpret.com or schedule a demo.